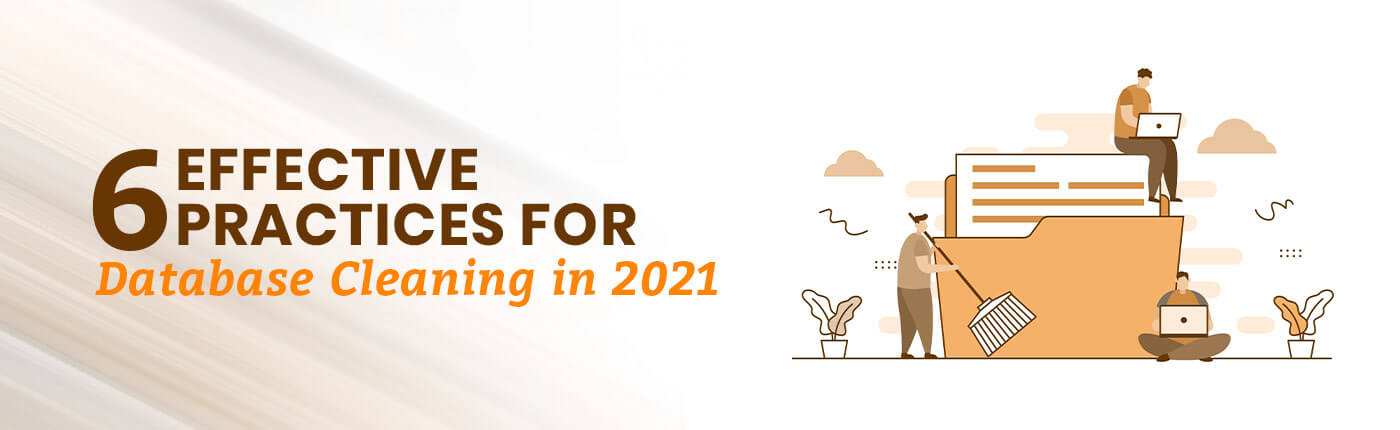
Every business adores its data. Gathering data is must for companies that want to unveil the insights with data analytic.
We have witnessed the massive rise of data in volume and variety of collected data.
Many business leaders know that data science applications can get the substantial value to the business. For instance, a business equipped with reliable and sophisticated database and CRM platforms can measure and understand the customer’s behavior at almost every stage of the buyer’s journey – this eventually translates to amelioration in sales and comprehensive customer interactions.
However, understanding the insights from the data is harder than it seems. Often these challenges arises from the poor quality data. And many companies use these data to support their business decisions, so it is necessary to ensure that the data is clean, reliable and good to use.
That’s where smart data cleaning best practices comes in.
Must Read: Data Hygiene: Mastering CRM Data Cleansing for Optimal Performance
Here are some Data cleansing best practices in 2024 which will help you maintain the sophisticated database.
1. Identify The Goal
You should know what your data should look like at the end stage, before initiating any data processing activities.
The vital key is to identify the objectives of your data cleaning strategy, rather working on solving the problems that will arise due to poor quality data .
Strategy I:
Identify the key objective of your work. What data you want to deal at the end stage.
Must Read: Benefits of Data Cleansing
2. Construct The Data Quality Criteria
What are your expectation for the data? You need to understand this foremost. Create Key Performance Indicators (KPIs) for the data quality.
Identify where most of the errors occurs and dig the root cause. Develop a plan, a robust strategy to ensure good health of your database.
Strategy II:
Know the KPI’s for your data quality.
Must Read: What Is Data Cleansing, Why Is It Important, And How Can You Do It?
3. Standard Operation Procedure (SOP)
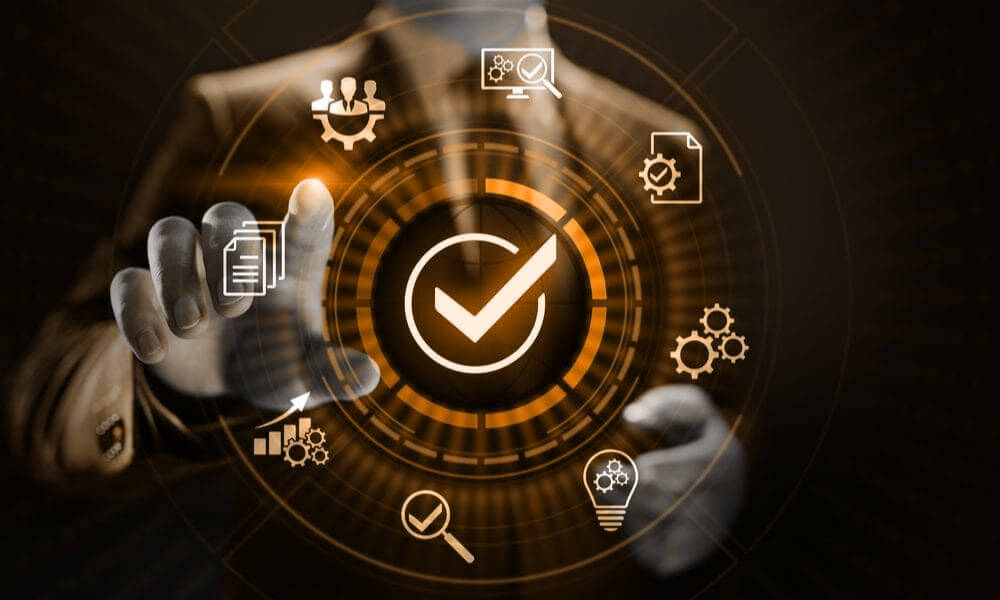
At the entry point itself if you check the important information it will help you with retaining the healthy data.
Following a standardized procedure will assist you to discern duplicates. While creating a database, remember to annex all the information after verifying and instruct the same to your team too. A sophisticated SOP will allow only quality data in the CRM.
Strategy III:
Create a standard procedure at the entry point.
Must Read: How Data Cleansing Help To Boost Your Marketing Campaign Performance?
4. Validate The Accuracy Level Of Data
Validate the data in real time. How? There are some competent tools such as Tibco Clarity, ZeroBounce and more. That offers information verification and ensures to deliver a seamless result.
Of course you can validate the information manually, but at the end human errors are inevitable. Also, manual validation would require a lot of time and may waste a lot of efforts if any error is passed unseen. So, better to take the help from the technology isn’t it?
Strategy IV:
Integrate tools to achieve maximum accuracy.
Must Read: What is CRM Data Cleansing?
5. Identify Duplicates
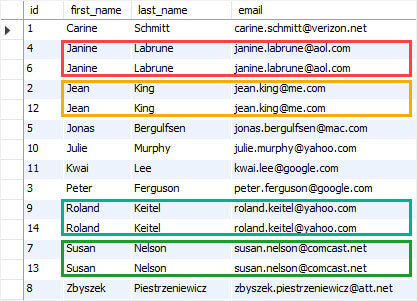
Source: MySQL Tutorials
Avoiding duplicates is the paramount of database cleansing. Duplicate data can make a business spend abundantly on general data maintenance and cause fallacious reports. To ensure this, first validate the data, scrub it to check duplicates and then remove.
Strategy V:
Never pass any duplicate data. Validate, Scrub, Delete, Repeat till you get an error free list.
Must Read: Everything You Need to Know About Automated Data Cleansing
6. Append Information
At this point you are having data that is standardized, validated, and scrubbed now you can combine it.
Since you have all the information just say – First Name, Last Name, Email and business address as the data record, ensure you do not miss to append, title, phone number revenue and ‘contact locations’ too.
Strategy VI:
Highlight the contact’s location, in order to abide the data protection and privacy laws.
Why care about Contact’s Location?
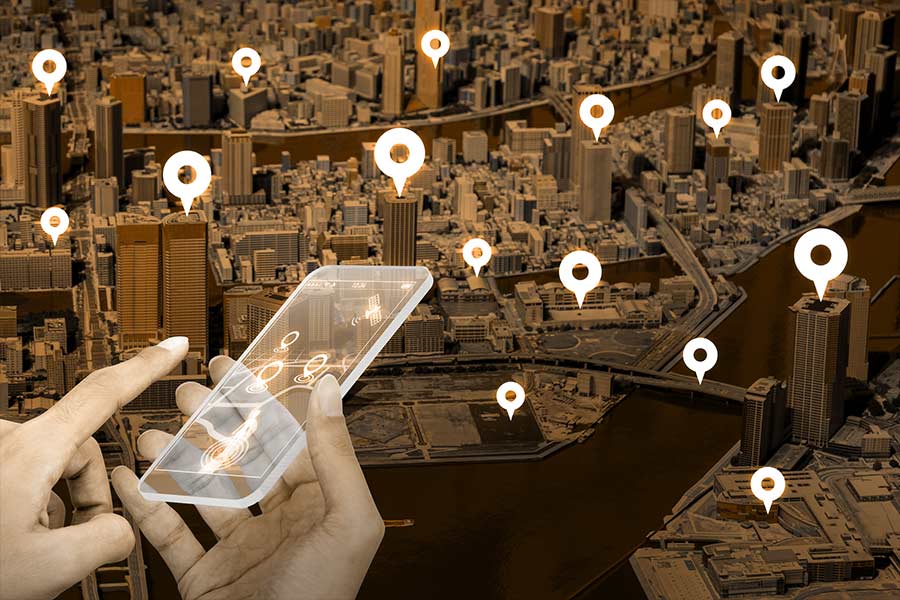
Because we must abide by the data protection and privacy laws and you should know it as early as possible so that you are clear which compliance rule to follow, whether GDPR or CASL.
If you are not having comprehensive data it means you have a ‘White Space’
There are many platforms which capture the accurate information about the contacts, like LinkedIn, you can compile it from such platforms.
Summary
Following the above mentioned Practices of Data Cleaning will help you with –
- Developing the strategy to strengthen the Database
- Standard process to ensure single customer view
- Rigorously follow the GDPR and CASL laws. Avoid any Compliance issues.
- Increase overall ROI by reducing overall budget wasted to retain the data.
As you start implementing and adopting the mentioned data cleansing best practices, you can surely expect the best return on your effort. Analyze and pinpoint the dirty data sources and begin with getting the good ROI.
Read Now: List Of Best 5 Database Cleansing Tools To Know
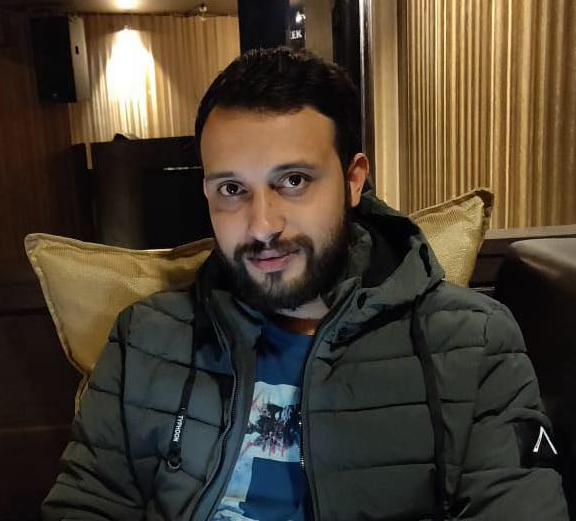
Vikas Bhatt is the Co-Founder of ONLY B2B, a premium B2B lead generation company that specializes in helping businesses achieve their growth objectives through targeted marketing & sales campaigns. With 10+ years of experience in the industry, Vikas has a deep understanding of the challenges faced by businesses today and has developed a unique approach to lead generation that has helped clients across a range of industries around the globe. As a thought leader in the B2B marketing community, ONLY B2B specializes in demand generation, content syndication, database services and more.