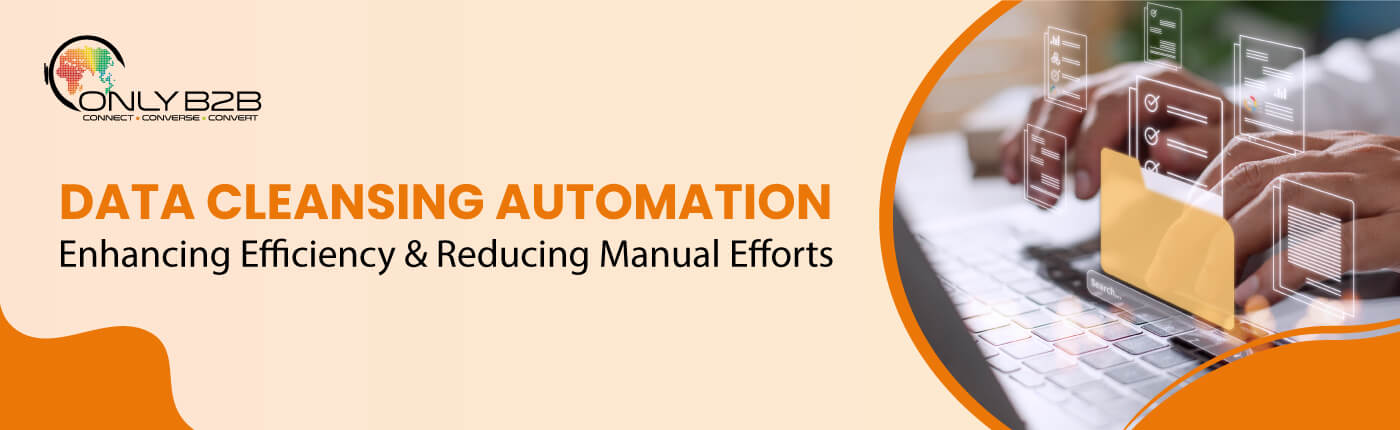
In the modern business environment, accurate and reliable data serve as the cornerstone of effective decision-making. Data cleansing, also known as data scrubbing or data cleaning, is the meticulous process of identifying and rectifying errors, inconsistencies, and inaccuracies within datasets. This practice is indispensable, as it ensures that the data on which critical decisions are based remains trustworthy and precise.
As the volume of data continues to surge with the advent of big data and analytics, maintaining data accuracy has become increasingly complex. Manual data cleansing processes that were once viable have become cumbersome and impractical due to the sheer magnitude and intricacy of data. Consequently, the demand for data cleansing automation has risen as organizations seek to streamline this vital process.
Data cleansing automation presents a spectrum of advantages beyond mere accuracy. Firstly, it significantly elevates data accuracy and quality. This precision is not just a luxury but a necessity; according to Gartner, organizations lose $15 million annually due to poor data quality. Moreover, automated data cleansing accelerates processes, leading to considerable time and cost savings.
Must Read: Data Hygiene: Mastering CRM Data Cleansing for Optimal Performance
Data Cleansing Automation: Enhancing Efficiency and Reducing Manual Efforts

Understanding Data Cleansing Automation
Data cleansing automation involves the utilization of advanced technologies, including algorithms, machine learning, and artificial intelligence, to autonomously detect and rectify errors within datasets. This approach not only heightens data accuracy but also minimizes the necessity for manual intervention.
A. Key Data Hygiene Challenges Faced by Businesses
The challenges of data hygiene, such as duplicate entries, inconsistent formatting, and incomplete information, hinder operational efficiency and decision-making. Manual addressing of these challenges has become impracticable, necessitating automated solutions that can handle the intricacies of modern data.
B. Role of Automation in Streamlining the Data Cleansing Process
Automation expedites the data cleansing process by promptly identifying errors and inconsistencies across extensive datasets. This translates to a more efficient process, reduced human effort, and the assurance that the data utilized for analysis is both accurate and reliable.
Must Read: What Is Data Cleansing, Why Is It Important, And How Can You Do It?
Advantages of Automated Data Cleansing
A. Improved Data Accuracy and Quality
Automated data cleansing ensures a higher level of data accuracy and quality. This is essential for decision-making accuracy; in fact, businesses believe that 32% of their business decisions are based on data that might be incorrect.
B. Time and Cost Savings
The time and costs associated with manual data cleansing are substantial. Automation significantly reduces the time required for data cleansing, resulting in substantial cost savings. According to Gartner, organizations that actively invest in data quality can expect a 25% improvement in their data quality metrics within a year.
C. Eliminating Human Errors and Bias
Manual data cleansing is vulnerable to human errors and biases, introducing inaccuracies into datasets. Automation eliminates this risk, ensuring data consistency and adherence to industry standards. IBM estimates that poor data quality costs the US economy $3.1 trillion annually.
D. Enhanced Data Security and Compliance
Automated data cleansing tools adhere to data protection regulations and data privacy requirements. This is especially crucial with regulations like the GDPR, which imposes strict fines for data breaches and non-compliance.
Must Read: How Data Cleansing Help To Boost Your Marketing Campaign Performance?
Essential Tools for Automated Data Cleansing
A. Data Cleaning and Enrichment APIs
Application Programming Interfaces (APIs) play a pivotal role in automated data cleansing. These APIs facilitate seamless integration with external data sources, enabling the enrichment and refinement of datasets. According to a study by MuleSoft, 83% of IT decision-makers reported that integrating APIs improved the efficiency of their business processes.
B. Data Quality and Integration Platforms
Robust data quality and integration platforms provide a comprehensive solution for businesses seeking to streamline their data cleansing processes. These platforms offer a range of functionalities, including data assessment, cleansing, and integration. According to Experian, 88% of companies believe that their bottom-line is affected by inaccurate data, which can be mitigated by utilizing such platforms.
C. Machine Learning and AI-Based Cleansing Solutions
Machine learning algorithms play a pivotal role in automated data cleansing by identifying patterns and anomalies within datasets. The utilization of AI-based solutions enhances the efficiency of data cleansing processes. A Forbes survey revealed that 51% of businesses are using AI for data quality and cleansing, emphasizing the growing importance of these technologies.
D. Custom Scripting and Automation Tools
Custom scripting and automation tools offer tailored solutions for specific data cleansing needs. These tools provide flexibility and customization options, allowing organizations to address unique challenges efficiently. According to Deloitte, 66% of businesses are leveraging automation to reduce time spent on data cleansing processes.
Must Read: 6 Effective Practices For Database Cleaning In 2023
Implementing Data Cleansing Automation
A. Assessing Data Cleansing Needs and Goals
Before embarking on data cleansing automation, businesses must evaluate their data cleansing requirements and establish clear objectives. According to Experian, organizations with a mature approach to data quality enjoy an annual revenue growth rate that is 2.8 times higher than those with lower data quality maturity.
B. Selecting the Right Automation Tools for Your Business
The selection of automation tools should align with the organization’s data complexity, industry regulations, and long-term objectives. According to TDWI, 56% of organizations identify data quality tools as critical components of their data strategies.
C. Integrating Automated Cleansing into Existing Workflows
The integration of automated data cleansing into existing workflows requires a strategic approach. This integration should be seamless, ensuring minimal disruption to ongoing operations. According to Informatica, 75% of organizations believe data integration is important to their digital transformation efforts.
D. Training and Skill Development for Efficient Utilization
Efficient utilization of automated data cleansing tools demands adequate training and skill development among the workforces. Proper training ensures that teams can harness the full potential of these tools to enhance data accuracy and efficiency. According to LinkedIn, 94% of employees would stay at a company longer if it invested in their career development.
Must Read: List Of Best 5 Database Cleansing Tools To Know
Key Components of an Automated Data Cleansing Strategy
A. Data Profiling and Analysis
Effective data profiling and analysis form the foundation of an automated data cleansing strategy. These processes involve a comprehensive assessment of data sets to identify inconsistencies and anomalies. According to Gartner, by 2022, 60% of large and global enterprises will develop in-house data profiling capabilities to enhance data quality and governance.
B. Deduplication and Record Matching
Deduplication and record matching are essential steps in data cleansing to ensure data accuracy and integrity. Duplicate records can lead to incorrect insights and decisions. A study by Harvard Business Review found that data decays at a rate of 30% per year, underscoring the need for consistent deduplication efforts.
C. Standardization and Formatting
Standardization and formatting are critical for maintaining data consistency across diverse sources. Inconsistent formats can hinder accurate analysis and decision-making. According to Data Entry Outsourced, businesses can save up to 60% in operational costs through efficient data standardization.
D. Validating and Correcting Inaccurate Data
Automated validation and correction of inaccurate data entries minimize errors and enhance data accuracy. Inaccurate data can lead to financial losses and operational inefficiencies. IBM estimates that poor data quality costs the U.S. economy around $3.1 trillion annually.
E. Enrichment and Augmentation with External Data
Leveraging external data sources for enrichment and augmentation enhances the value of datasets. This external data can provide valuable context and insights for more informed decision-making. According to D&B, businesses that leverage external data sources experience 9% higher revenue growth.
Must Read: Database Cleansing – Importance And GDPR Compliance
Measuring the Success of Data Cleansing Automation
A. Tracking Data Quality Metrics
Monitoring data quality metrics, such as accuracy rates and completeness, provides tangible insights into the effectiveness of automated data cleansing efforts. Organizations can use these metrics to gauge improvements over time. According to Gartner, organizations that invest in data quality will see a 20% reduction in operational costs.
B. Assessing Reduction in Errors and Inconsistencies
Quantifying the reduction in errors and inconsistencies achieved through automated data cleansing underscores the impact of automation on data accuracy. This reduction directly translates into improved decision-making and operational efficiency. According to Experian, 27% of businesses believe data quality is the most significant obstacle to B2B lead generation.
C. Measuring Efficiency Gains and Time Savings
Efficiency gains and time savings resulting from automated data cleansing processes are significant indicators of success. Automation accelerates the data cleansing process, allowing resources to be allocated to more strategic tasks. A study by McKinsey found that companies that use data effectively are 23 times more likely to acquire customers.
Must Read: Automating B2B List Building: Tools and Techniques for Streamlined Lead Generation
Overcoming Challenges in Data Cleansing Automation
A. Dealing with Complex Data Structures and Sources
Automation tools must be adaptable to handle diverse data structures and sources. As organizations collect data from various channels, the complexity of data structures increases. According to Deloitte, 60% of surveyed organizations struggle with complex data structures. Data cleansing automation solutions should be versatile enough to accommodate this diversity.
B. Handling Large Volumes of Data
The era of big data has brought about immense challenges in handling large volumes of data. Automation solutions need to be scalable to manage and process extensive datasets efficiently. IDC predicts that the global datasphere will grow from 33 zettabytes in 2018 to 175 zettabytes by 2025, highlighting the need for scalable data cleansing solutions.
C. Addressing Privacy and Data Security Concerns
With the rising emphasis on data privacy and security, automated data cleansing tools must prioritize these concerns. Regulations like GDPR and CCPA impose strict data protection standards. According to the Ponemon Institute, the global average cost of a data breach is $3.86 million. Data cleansing automation tools must adhere to these standards to mitigate the risk of breaches.
Must Read: What Is Database Cleansing and Why Is It Important?
Future Trends in Data Cleansing Automation
A. Advancements in AI and Machine Learning
The future of data cleansing automation is intertwined with advancements in artificial intelligence and machine learning. AI algorithms can learn from patterns in data and make accurate decisions, enhancing the efficiency of data cleansing. IDC predicts that spending on AI and ML will reach $97.9 billion by 2023.
B. Integration with Big Data and Cloud Technologies
Data cleansing automation will seamlessly integrate with big data and cloud technologies. This integration ensures that data cleansing processes can handle the vast amounts of data generated and stored in cloud environments. According to Gartner, by 2022, public cloud services will be essential for 90% of data and analytics innovation.
C. Predictive Data Cleansing for Proactive Data Maintenance
The future will witness the evolution of predictive data cleansing, where organizations will identify potential data issues before they disrupt operations. Predictive analytics will play a pivotal role in proactively addressing data quality concerns. Research by Forbes Insights found that 86% of senior executives believe data quality is crucial for predictive analytics success.
Must Read: Top 9 Benefits Of Data Cleansing That Will Blow Your Mind
Conclusion
In conclusion, data cleansing automation is a transformative approach that enhances efficiency and reduces the burden of manual efforts. As businesses continue to gather vast volumes of data, the importance of accurate and reliable information cannot be overstated. Automated data cleansing empowers organizations to make informed decisions, reduce errors, save time, and maintain data security and compliance.
The benefits of data cleansing automation span improved data accuracy, significant time and cost savings, elimination of human errors and bias, and heightened data security and compliance. Implementing automation requires a strategic approach, from assessing data cleansing needs to selecting the right tools and integrating them seamlessly into existing workflows. The success of automation is measurable through data quality metrics, reduction in errors, and efficiency gains.
However, challenges such as complex data structures, large data volumes, and data privacy concerns need to be addressed. The future of data cleansing automation holds promise with advancements in AI and machine learning, integration with cloud technologies, and the emergence of predictive data cleansing for proactive data maintenance.
As businesses navigate the data-driven landscape, embracing automation for data hygiene is not just a necessity but a strategic advantage. By investing in automated data cleansing solutions, organizations can unlock the full potential of their data, driving more accurate insights, informed decision-making, and a competitive edge in a data-driven world.
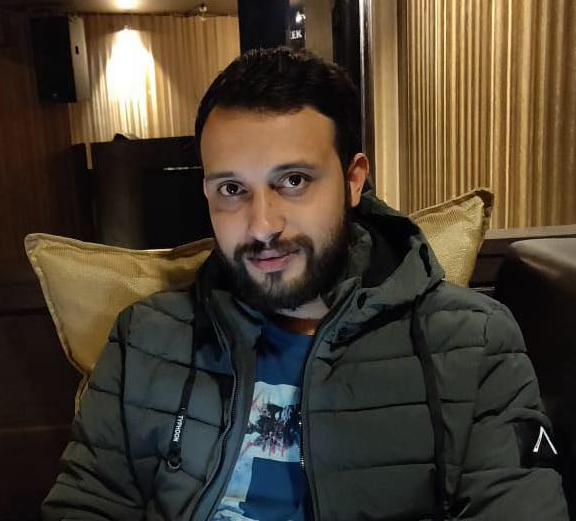
Vikas Bhatt is the Co-Founder of ONLY B2B, a premium B2B lead generation company that specializes in helping businesses achieve their growth objectives through targeted marketing & sales campaigns. With 10+ years of experience in the industry, Vikas has a deep understanding of the challenges faced by businesses today and has developed a unique approach to lead generation that has helped clients across a range of industries around the globe. As a thought leader in the B2B marketing community, ONLY B2B specializes in demand generation, content syndication, database services and more.