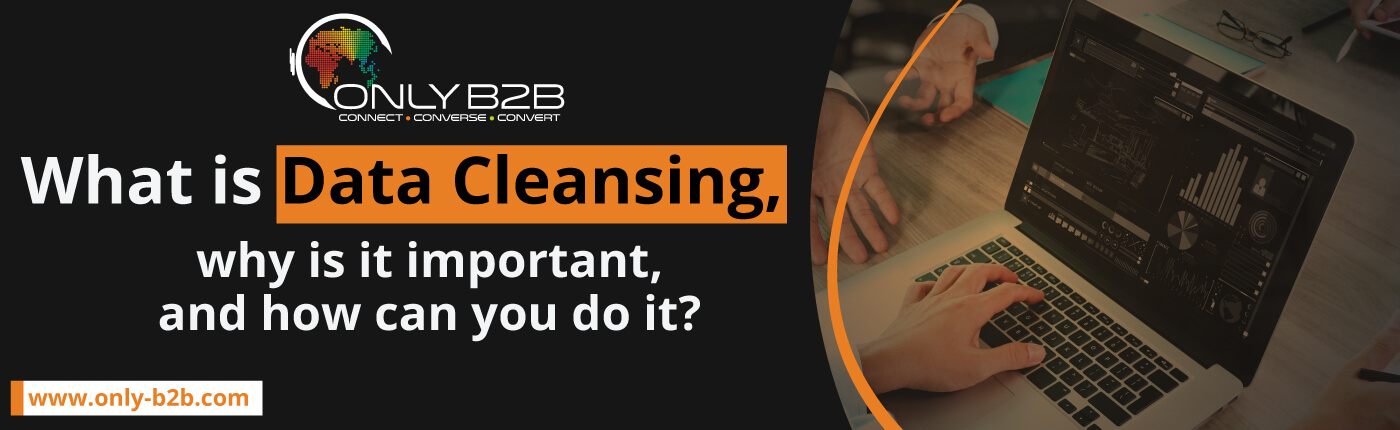
One of the most crucial processes in the data preparation process is data cleansing and purification. As businesses depend more and more on data to guide important choices, poor data can result in inefficiencies, missed opportunities, or even monetary losses. Therefore, one of the largest challenges facing organizations today is maintaining a “clean” database. The importance of data cleansing cannot be emphasized enough, as it ensures the integrity and reliability of the information upon which significant choices hinge.
What is Data Cleansing? And Why is It Important?
Table of Contents
- 1 What is Data Cleansing? And Why is It Important?
- 2 What Different Kinds Of Data Issues Are There?
Data preparation starts with data cleansing, sometimes referred to as data scrubbing or data cleaning. Simply said, data cleansing is the process of identifying and deleting any inaccurate, incomplete, or irrelevant data from the data set. Data cleansing can be carried either manually or with the use of software.
Must Read: CRM Data Cleansing for Optimal Performance
What Different Kinds Of Data Issues Are There?
When businesses obtain data from the internet or other sources, merge data from several data sets, acquire data from clients or other departments, etc., a number of issues related to data quality can arise. These issues underscore the importance of data cleansing, as ensuring clean and accurate data becomes crucial in mitigating challenges.
Typical issues include:
1. Duplicate Data:
Two or more records that are exactly the same.
2. Conflicting Data:
When different facts are contained in the same record. As an instance, consider a customer with the same name but distinct phone numbers in several records.
3. Incomplete Data:
Data that have missing characteristics.
4. Invalid Data:
Data that does not comply to guidelines.
The Risk Posed By “Poor Data”
Data is now one of the most valuable assets for the majority of businesses worldwide. Poor data can hurt a firm’s bottom line as more individuals begin to rely on their data to make important decisions. Indeed, according to Forbes, firms are losing up to 12% of their revenue due to bad data, and in the United States alone, dirty data is estimated to cost the economy $3.1 trillion annually.
Poor data not only hurts businesses financially, but also wastes a lot of time because data analysts spend more than half of their time monitoring and cleaning data. The company as a whole will move more slowly as a result of this added time.
Additionally, incorrect data might have an impact on a variety of other business operations. For instance, insufficient information on client preferences may result in unproductive marketing initiatives, and inaccurate customer data may cause issues for sales.
This underscores the critical question: why is data cleansing important? Ensuring the cleanliness and accuracy of data becomes imperative to prevent such substantial financial losses and to maintain the trustworthiness of the information driving key decisions.
Must Read : 6 Effective Practices For Database Cleaning
Advantages Of Data Cleansing
Several advantages of data cleansing include:
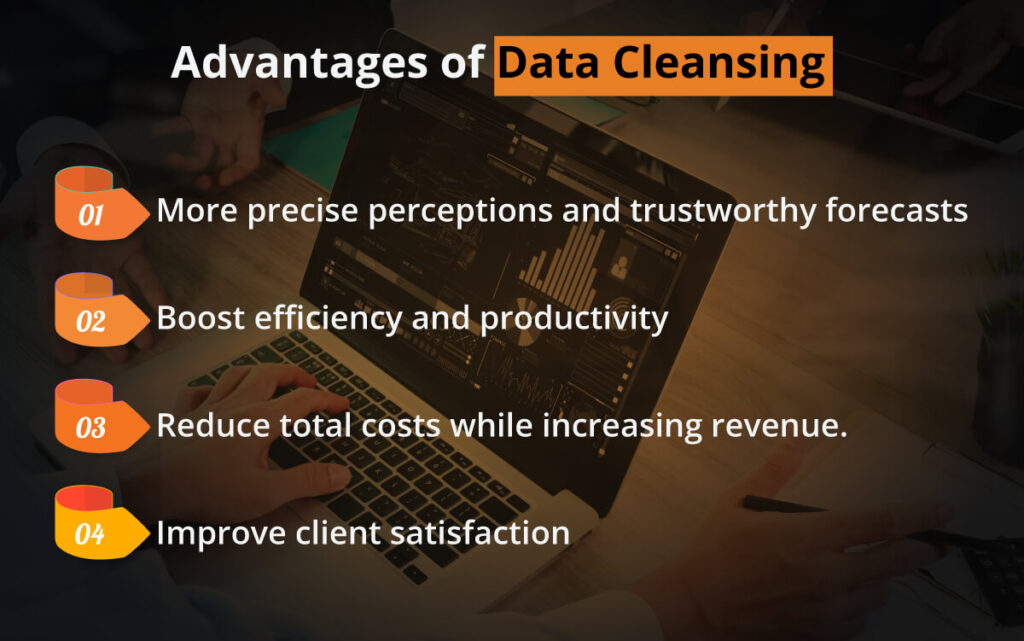
1.More Precise Perceptions And Trustworthy Forecasts:
Data information will be more trustworthy if better data is available for processing. The business will gain insights into several fields as a result, which will aid in making more precise predictions.
2. Boost Efficiency And Productivity:
Dirty data can cause problems, work-to-be-done issues, and bottlenecks in a variety of processes. By removing this barrier, employees can do their tasks more quickly and proficiently. And it Helps To Boost Your Marketing Campaign Performance.
3. Reduce Total Costs While Increasing Revenue:
According to research, dirty data can account for up to 12% of a company’s revenue losses. Effective data cleansing can minimize this loss and increase the company’s overall revenue.
4. Improve Client Satisfaction:
Businesses may better understand their customers by using more precise data, which will improve the overall customer experience.
Best Methods For Data Cleansing:
There are several methods and procedures for maintaining a neat database. Here are some recommendations for data cleansing.
1. Create A Data Quality Plan:
- Establish goals for your data.
- Create key performance indicators (KPIs) for data quality. What are they and how will you achieve them? How will you keep tabs on the condition of your data? How will you consistently manage data hygiene?
- Learn where the majority of data quality issues arise.
- Recognize false data.
- Recognize the underlying cause of the data issue.
- Create a strategy for maintaining the integrity of your data.
2.Valid Data At The Entry Point:
To maintain a clean database, it’s critical to have clean, standard data that guarantees all crucial properties are free of errors at the time of input. Before continuing, this can help your team save time and effort.
The entire team should establish and follow a standard operating procedure for entering data. This will guarantee that the system can only accept high-quality data.
3.Verify Your Data’s Accuracy:
A small data collection can be manually validated at this step to ensure that the data satisfies all of the requirements. The manual technique is time-consuming, labor-intensive, and inefficient with larger and more complicated data sets since people are prone to making mistakes. Tools for data quality control are therefore created to assist with this problem.
4. Deal With Duplication:
Duplicates consume time and effort and are detrimental. They slow down the business’s operations, harm company-customer relationships, and interfere with a number of company operations, including marketing, sales, and customer support.
Companies should take every precaution to avoid them. It’s also critical to take into account the following after eliminating all duplicate data at the entrance:
- Data conversion to a single format for processing and analysis is known as bastardization.
- Making sure all data is recorded consistently is known as normalization.
- The act of merging involves joining pertinent portions of various datasets that contain data that is dispersed across them in order to produce a single file.
- Data sorting and summarization are both considered to be aggregate actions.
- Filtering is a process of reducing a dataset such that it only contains the data that consumers are interested in.
- Scaling is the process of transforming data to match a given range, such as 0-100 or 0-1.
- To avoid a poor fit in linear regression, remove duplicate and outlier data points.
5. Add Missing Information:
The process of appending involves adding missing data to entries in mandatory fields, such as phone numbers, email addresses, last and first names, Office Address, etc. Finding the missing details, though, might be challenging. It is advised that businesses employ a trustworthy third-party data source to help fill in the gaps in order to complete this phase successfully.
6. Encourage The Organization As A Whole To Use Clean Data:
After everything is finished, you must inform everyone within the organization of the significance of clean data. Make sure all staff members, irrespective of their functions, are aware of and uphold the practice of clean data.
At Only B2B, we identify practical answers to business issues, use a consultative approach to every client engagement, and help your company achieve the best possible business results. Talk to us and explore the various demand generation services we have in store for you today!
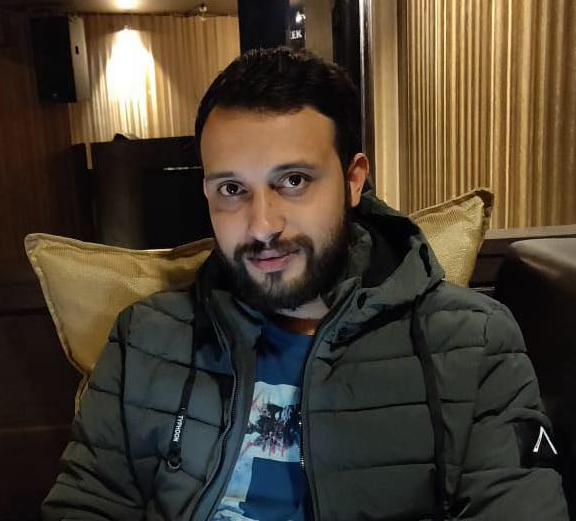
Vikas Bhatt is the Co-Founder of ONLY B2B, a premium B2B lead generation company that specializes in helping businesses achieve their growth objectives through targeted marketing & sales campaigns. With 10+ years of experience in the industry, Vikas has a deep understanding of the challenges faced by businesses today and has developed a unique approach to lead generation that has helped clients across a range of industries around the globe. As a thought leader in the B2B marketing community, ONLY B2B specializes in demand generation, content syndication, database services and more.